Is pedigree low quality
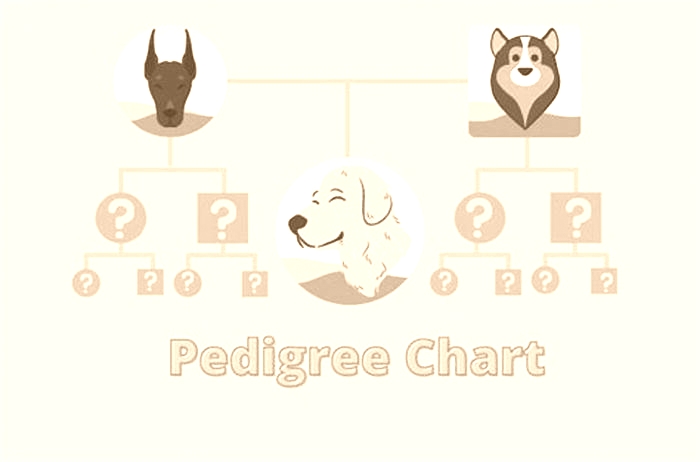
Pedigree Dog Food Review (2024)
Pedigree Dog Food, a well-known brand in the pet nutrition space, lists several key components as the foundation of its recipes. These ingredients include ground whole corn, animal fat, and beet pulp, alongside ground whole wheat, meat and bone meal, and chicken by-product meal. Additional elements such as vegetable oil, brewers rice, corn gluten meal, and an array of vitamins and minerals are also integral to the formulation, designed to meet the nutritional needs of dogs.
However, the inclusion of artificial colors and preservatives in Pedigree Dog Food raises concerns regarding its suitability for all pets, especially those with sensitive systems. The prominence of grain-based ingredients, such as corn and wheat, may also present challenges for dogs prone to food allergies or gluten sensitivities, potentially limiting the foods applicability to a broader canine demographic. With a protein content hovering around 22%, the food offers a standard level of protein that is typical for dry dog foods but may not meet the higher protein requirements of some dogs, particularly those with active lifestyles or specific dietary needs.
A detailed examination of Pedigree Dog Foods ingredient list brings to light some critical considerations for pet owners. The reliance on grains as primary ingredients, while economically efficient, might not align with the dietary preferences or health requirements of all dogs. Similarly, the use of artificial additives, while common in many pet foods, is increasingly becoming a point of contention among pet owners who prefer natural and wholesome diets for their companions.
Given these factors, it is essential for pet owners to evaluate Pedigree Dog Foods compatibility with their pets unique health profiles and dietary restrictions. While Pedigree offers a convenient and accessible option for many, the decision to choose this brand should be informed by a careful consideration of its ingredients and how they align with the nutritional philosophy and needs of each individual dog. As pet nutrition continues to evolve, the demand for transparency, quality, and adaptability in dog food ingredients becomes paramount in selecting the best diet for our furry family members.
Pedigree Dog FoodProduct Line
Pedigree, a prominent name in the world of pet nutrition, boasts an extensive selection of dog food that caters to the diverse needs and preferences of canine companions. Available for purchase online, the brand offers an impressive lineup of over 60 varieties of dry and wet dog food, ensuring that dog owners can find the perfect match for their furry friends dietary requirements. This wide range of options underlines Pedigrees commitment to providing comprehensive nutrition solutions for dogs of all ages, sizes, and breeds.
Upon examining the ingredient lists across various Pedigree products, one might observe a pattern of similarity not only within the Pedigree brand but also among other dog food brands under the Mars umbrella. This resemblance in ingredient composition reflects a consistent approach to pet nutrition across the companys brand portfolio. While the variety of Pedigrees offerings is vast, the foundational ingredients used in many of their formulations tend to mirror each other, showcasing a uniform strategy in dietary formulation that Mars applies to its pet food products.
The extensive array of Pedigree dog foods, despite the ingredient similarities, is designed to meet specific nutritional needs and preferences. From specialized formulas aimed at supporting joint health, weight management, and digestive wellness to products tailored for puppies, adults, and senior dogs, Pedigree strives to address the unique nutritional demands of dogs at different life stages and with varying health considerations.
11 Varieties of Pedigree Dry Dog Food
53 Varieties of Pedigree Wet Dog Food
Pedigree Recall History
Over the past decade, Pedigree, like many pet food brands, has experienced a few recalls due to various concerns. Heres a summary of the notable recalls affecting Pedigree Dog Food within this timeframe:
- June 2012 Recall: Pedigree issued a voluntary recall for three varieties of their weight management canned dog food products due to potential choking hazards. The recall was initiated after reports of small pieces of blue plastic found in the food, which resulted from a manufacturing error.
- August 2014 Recall: This recall involved specific bags of Pedigree Adult Complete Nutrition dry dog food sold in Dollar General stores across four U.S. states. The reason for the recall was the potential presence of metal fragments. Although no health issues were reported, the recall was a precautionary measure to prevent the risk of injury to pets.
Dog Food Network Score
Score: 3.5/10
Reasoning:
- Ingredient Quality:Pedigree often relies heavily on grain-based carbohydrates such as corn and wheat,which are less bioavailable sources of protein for dogs compared to high-quality meats.They also often utilize meat by-products and other less desirable protein sources.
- Recall History:Pedigrees recall history isnt terrible,but it does include potential contamination concerns on a few occasions,raising some flags compared to brands with cleaner records.
- Reviews:Pedigree gets very mixed reviews.Some consumers report their dogs enjoy it and seem to do well long-term.However,many criticize the ingredients and express concerns about its long-term impact on their pets health.
- Popularity:Pedigree is one of the best-selling dog food brands worldwide due to its widespread availability and low price point.
Why a 3.5 and Not Lower:
While I find Pedigree lacking in nutritional quality, certain factors justify it staying above a rock-bottom score:
- Affordability:Budget is a huge consideration for many pet owners and Pedigree delivers in that arena.
- Variety:Pedigree offers a massive range of wet and dry formulas,accommodating varied preferences and even some age-specific diets.
- Accessibility:You can find Pedigree virtually everywhere,making it convenient in an emergency situation.
Overall:
Pedigree serves a clear purpose in the market. Its popularity stems from its low price and availability. However, quality issues raise valid concerns when choosing a primary food source for your dog. If on a tight budget, Pedigree may not be the worst option, but pet owners who prioritize premium ingredients and long-term health may want to consider alternatives if feasible.
Improved computations for relationship inference using low-coverage sequencing data
In Sects.2.2 through2.6 we will define a method that, given lcNGS data for two or more individuals and two alternative pedigrees relating them, computes a likelihood ratio. Combining this ratio with a prior odds yields a posterior odds which may be used to choose between the pedigrees. To assess such a method, the best alternative is of course to apply it and competing methods to large numbers of cases where the true pedigree is known in each case. Such comparisons are limited by the availability of data.
An alternative, used in this paper, is to compare competing methods on simulated data. The assessment is then divided into two tasks: Showing that the simulation model yields data that is realistic in relevant ways, and comparing methods on the simulated data. The simulation model used in this paper uses population models and inheritance models presented in Sects.2.3 and2.4, where the inheritance model includes linkage (i.e., effects of crossovers inside the considered pedigree). When two loci are strongly linked, their alleles will often be inherited together, as haplotypes, through the pedigree, thus strongly influencing the information about the pedigree contained in the data. This motivates why data simulation should contain linkage.
The population model includes important standard features such as kinship, however, it does not include linkage disequilibrium (LD, i.e., effects of crossovers outside of the considered pedigree). This means that the effect of LD on competing methods is not assessed. Current methods for handling LD include grouping markers together [9] or using an multiorder Markov chain [19]. Both ideas may be possible to combine with our approach. We have chosen to defer treatment of LD to a later paper.
Section2.2 presents the observational model we use to simulate lcNGS data from simulated genotypes. This is a simplified model simulating only counts of reads at each locus. Section3.1 contains a small study and an argument why we believe this observational model captures features of lcNGS data essential for relationship inference, in particular when one or more of the samples are based on small amounts of DNA.
Our likelihood method for pedigree inference uses exactly the same likelihood as the one used in data simulation. In any simulation study, when simulation is done using a particular probability distribution, it will be optimal to use the same distribution for likelihood computations. What our study illustrates is the size of the performance reduction when using a likelihood method that ignores linkage or the uncertainty in genotypes that is inherent in lcNGS data. Finally, we compare our approach with NgsRelate [18] which uses a maximum likelihood procedure to find the most likely Jacquard coefficients. NgsRelate does not account for genetic linkage between the included genetic markers.
Model overview
We assume we have data concerning N autosomal loci for T tested persons. For each considered pedigree we would like to compute the probability of the observed data given the pedigree. We model this probability using a population model including an (F_{st}) adjustment [20] but no linkage disequilibrium (LD), an inheritance model including a Poisson crossover model but no mutations, and an observational model featuring reads that are sampled from true alleles with some possibilities for errors. The text below is formulated in terms of markers with at most four alleles, which is the nature of SNPs. However, the model is not per se restricted to tetra-allelic data.
Observational model
At a given genetic locus for a tested person, we assume the data consists of a vector (c=(c_1,c_2,c_3,c_4)) of counts of reads corresponding to nucleotides A, C, G, T, respectively. Assume the true genotype at this locus is (g=(g_1,g_2)), coded as two indices between 1 and 4. We describe the relationship between c and g as a result of two separate stochastic events: First, the proportion q of DNA segments after PCR that are based on (g_1) among those based on either (g_1) or (g_2) is modelled as (q=k/m) where (ksim {text {Binomial}}(m,1/2)). Here m is an integer parameter connected to the sample, representing the approximate number of DNA templates from the sample that end up founding PCR amplicons for this locus. For high m, we have (qapprox 1/2), while for lower m, q can be close or even equal to 0 or 1, meaning that one of the alleles failed to be picked up in the PCR process.
Writing (C=c_1+c_2+c_3+c_4) and (beta =(beta _1,beta _2,beta _3,beta _4)), we model (cmid q, g sim {text {Multinomial}}left( C, beta right)) where for (i=1,2,3,4),
$$begin{aligned} beta _i = left( 1-eright) (qI(g_1=i) + (1-q)I(g_2=i)) + frac{e}{4} end{aligned}$$
(1)
where e is a small positive model parameterFootnote 1 relating to e.g. sequencing or mapping errors. In other words, for each observation of a read, there is a small probability e that it is in fact unrelated to the underlying genotypes, and the probability is then 1/4 for reporting each genotype. With probability (1-e), the read is based on (g_1) with probability q and on (g_2) with probability (1-q). Putting the two stochastic events together we get that
$$begin{aligned} {text {Pr}}(cmid g) = sum _{k=0}^m{text {Pr}}(cmid q=k/m, g)left( {begin{array}{c}m\ kend{array}}right) 2^{-m}. end{aligned}$$
(2)
In Sect.3.1 we compare calculated probabilities from the model above with real data to argue that the two parameters m and e in our model can capture the most important features of variability in observational data. We note that low quality and quantity DNA samples can be modelled with a low m (sometimes in the range 510), since few and damaged DNA molecules is directly correlated to a low m. On the other hand, e, usually attributed to sequencing or mapping errors, is generally low (quite close to zero) with modern sequencing and bioinformatic tools [21].
To make likelihood computations for case sample data with the model above, a user has to provide information about the parameters m and e. One possibility is to use the results of Sect.3.1 to select values. Alternatively a user may provide priors: If M possible values (m_1,m_2,dots ,m_M) with probabilities (p_1,p_2,dots ,p_M) approximately describes prior knowledge about the parameter m, and similarly E possible values (e_1,dots ,e_E) with probabilities (q_1,dots ,q_E) describes a prior for the parameter e, then we may compute
$$begin{aligned} {text {Pr}}(text {data}mid text {pedigree}) = sum _{i=1}^Msum _{j=1}^E{text {Pr}}(text {data}mid text {pedigree},m_i,e_j)p_iq_j. end{aligned}$$
(3)
Population model
We assume population independence between different loci, i.e. there is no association (linkage disequilibrium, LD) between alleles at different loci. At a locus, assume there are F founding alleles, so that the remaining alleles in the pedigree are inherited from these. We provide a stochastic model for the vector (h=(h_1,h_2,h_3,h_4)) of counts of how many of the F founding alleles are of each of the four possible types. Assume the population frequencies of the four possible alleles are (f=(f_1,f_2,f_3,f_4)). We then model
$$begin{aligned} hmid f,theta ,gamma sim {text {Dirichlet-Multinomial}}left( F, left( 1/theta -1right) left[ f(1-gamma )+gamma {overline{f}}right] right) . end{aligned}$$
(4)
Here, (theta) is the kinship parameter (population fixation parameter) (F_{st}) [20] while (gamma) is a small positive number and ({overline{f}}) is a vector with general probabilities for observing A, C, G, or T for any of the markers. Note that with this model, after having observed k alleles of type i and (s-k) alleles of other types, the probability for observing another allele of type i is
$$begin{aligned} frac{k + (1/theta -1)(f_i(1-gamma )+gamma {overline{f}}_i)}{s + 1/theta -1 } = frac{theta k + (1-theta )(f_i(1-gamma )+gamma {overline{f}}_i)}{1 + theta (s-1)}. end{aligned}$$
(5)
Note also that Eq.5 reduces to the traditional (F_{st}) formula [20] when (gamma =0).
To motivate why one might use a number larger than zero for (gamma), consider the following example: We compute the likelihood ratio comparing the alternatives of two persons being unrelated or half-siblings using data at a single locus. Assume data for both persons contains 50 reads indicating nucleotide G, while the population frequency of G is zero. With (gamma =0), all reads for G will be attributed to read errors (which may seem unreasonable) and the likelihood ratio would be approximately 1. With (gamma) some small positive number, the likelihood ratio in favour of unrelatedness would instead be approximately equal to the probability of observing a second G allele among the founder alleles of the case data after having observed a first G allele, which in our model would be
$$begin{aligned} theta + (1-theta )gamma {overline{f}}_3. end{aligned}$$
(6)
Finally, note that our model can be interpreted as using frequencies from a database of size ((1/theta -1)(1-gamma )) and a pseudo-count vector ((1/theta -1)gamma {overline{f}}).
Inheritance model
Given a pedigree consisting of K parentchild relationships. Consider the set ({{mathcal {A}}}) consisting of vectors r of length K where each component is either 0 or 1, with 0 indicating that the child in the corresponding relationship has inherited the parents maternal allele, while 1 indicates inheritance of the paternal allele. We call such a vector an inheritance pattern. Each value of r organizes the alleles of the persons in the pedigree into subsets of alleles that must be identical as long as we disregard mutations, which is reasonable to do for SNPs. Restricting ourselves to the typed persons, we represent such a partition as a vector of length 2T of subset indices, with each of the T pairs representing the maternal and paternal alleles of a person. We enumerate the subsets using consecutive integers starting from zero. For any two subsets, if there exists one or more persons in which alleles from exactly one of the subsets occur, consider the first person, in a fixed ordering of the persons, in which this happens, and the subset with an allele in this person. This subset will then be indexed with a lower integer compared to the other subset. Finally, for each pair of integers representing the alleles of a single person, if the first integer is larger than the second, we switch the two integers. This creates a unique code for each partitioning of unordered pairs of alleles: We call this an IBD code.
As an example, consider a nephew and his paternal uncle. We get two possible IBD codes (0,1,2,3) and (0,1,0,2). As the pedigree may be defined by (K=5) relationships, we have that the corresponding r vector has (2^5=32) possible values. Each of these values map to one of the IBD codes above. If we assign equal probability to each of the possible r vectors, the induced probabilities on the two IBD codes above are both 0.5.
Let us now write (r_i) for the inheritance pattern at locus i. Let h(r) denote the IBD code for an (rin {{mathcal {A}}}). Given the IBD code for a locus and the observational and population models above, we may compute the probability of the observed data at the locus by conditioning on and summing over all possible combinations of alleles for the subsets indicated by the IBD code. Write (L_i(h(r_i))) for the probabilityFootnote 2 of the data at locus i. The functions (L_i) are determined by our observational and population models. The complete model probability can now be written
$$begin{aligned} {text {Pr}}(text {data}mid text {pedigree}) = sum _{(r_1,dots ,r_N)}{text {Pr}}(r_1,dots ,r_N)prod _{i=1}^NL_i(h(r_i)) end{aligned}$$
(7)
where we sum over all possible inheritance patterns for all loci.
It remains to specify the joint probability model for the vectors (r_1,r_2,dots ,r_N). To simplify we assume a Markov model so that each (r_{i+1}) is independent of (r_1,dots ,r_{i-1}) given (r_i). Specifically, we assume there is a given probability (p_i) for an odd number of crossovers between locus i and (i+1) independently for all relationships defining the pedigree. This yields the conditional probabilities
$$begin{aligned} T_i(r_i,r_{i+1}) overset{text {def}}{=}{text {Pr}}(r_{i+1}mid r_i) = prod _{j=1}^Kp_i^{I(r_{ij}ne r_{i+1,j})} (1-p_i)^{I(r_{ij}=r_{i+1,j})}, end{aligned}$$
(8)
where we write (r_i=(r_{i1},r_{i2},dots ,r_{iK})) and (r_{i+1}=(r_{i+1,1},r_{i+1,2},dots ,r_{i+1,K})). The Markov assumption together with a uniform probability on (r_1) now yields a joint probability model for (r_1,r_2,dots ,r_N).
A possible computational algorithm
The Markov assumption above makes it possible to use an iterative algorithm to compute the value of Eq.7, in fact, a version of the Lander-Green algorithm [10]. Specifically, let us write (d_i) for the data at locus i and suppress the pedigree from the notation. For (i=1,dots ,N) and all values of (r_i), we may compute
$$begin{aligned} {text {Pr}}(d_1,dots ,d_i,r_i) = {text {Pr}}(d_1,dots ,d_{i-1},r_i) {text {Pr}}(d_imid r_i) end{aligned}$$
(9)
and (for (i<N))
$$begin{aligned} {text {Pr}}(d_1,dots ,d_i,r_{i+1}) =sum _{r_i} {text {Pr}}(d_1,dots ,d_i,r_i) {text {Pr}}(r_{i+1}mid r_i). end{aligned}$$
(10)
Noting that ({text {Pr}}(d_imid r_i)=L_i(h(r_i))), that ({text {Pr}}(r_{i+1}mid r_i)=T_i(r_i, r_{i+1})), and that
$$begin{aligned} {text {Pr}}(text {data}mid text {pedigree}) = {text {Pr}}(d_1,dots ,d_N) = sum _{r_N}{text {Pr}}(d_1,dots ,d_N,r_N), end{aligned}$$
(11)
we obtain the following algorithm:
Initialize a vector z of length (2^K) with values (1/2^K), so that it represents the prior probability distribution on (r_1). Then, for each locus (i=1,dots ,N):
- 1
Compute a vector of length (2^K) by computing (L_i(h(r_i))) for all possible values of (r_i). Multiply it term-wise with z to get a vector representing ({text {Pr}}(d_1,dots ,d_i,r_i)) for all possible values of (r_i).
- 2
If (i<N), compute a matrix M of size (2^Ktimes 2^K) representing (T_i(r_i,r_{i+1})) for all possible values of (r_i) and (r_{i+1}). Then set z equal to the matrix product zM, so that z now represents ({text {Pr}}(d_1,dots ,d_i,r_{i+1})).
Finally, sum the elements of z to obtain the probability we wanted to compute.
The computed number will in real examples be extremely small, so that one needs to compute its logarithm to avoid numerical underflow. In practice we re-scale the values in z in every loop above, storing separately the logarithm of a common factor.
An improved algorithm using symmetries
An important problem with the algorithm above is that (2^K) can be a large number even for fairly small K, so that the ((2^Ktimes 2^K)) matrix M representing (T_i(r_i,r_{i+1})) can become too large to handle. However, it turns out that in practice the vector z will contain many repeated values. This opens up the possibility of using a compressed matrix (M^*) for computations.
Specifically, consider a subdivision
$$begin{aligned} {{mathcal {A}}}= & {} {v_{11},v_{12},dots ,v_{1n_1}}cup {v_{21},v_{22},dots ,v_{2n_2}}cup dots nonumber \{} & {} dots cup {v_{J1},v_{J2},dots ,v_{Jn_J}} end{aligned}$$
(12)
of the set of inheritance patterns ({{mathcal {A}}}) into disjoint subsets where for all v and (v^*) in a common subset there exists a permutation g on ({{mathcal {A}}}) so that (1) for all (rin {{mathcal {A}}}) (h(g(r))=h(r)), (2) for all (r,r'in {{mathcal {A}}}) g(r) and (g(r')) differ in the same number of components as r and (r'), and (3) (g(v)=v^*). Then we will show in the Additional file 1 Appendix that
$$begin{aligned} {text {Pr}}(d_1,dots ,d_i,r_i=v) = {text {Pr}}(d_1,dots ,d_i,r_i=v^*) end{aligned}$$
(13)
and that for all (i=1,dots ,N-1) and (k=1,dots ,J)
$$begin{aligned} {text {Pr}}(d_1,dots ,d_i,r_{i+1}=v_{k1}) =sum _{j=1}^J{text {Pr}}(d_1,dots ,d_i,r_i=v_{j1}) left( sum _{s=1}^{n_j}T_i(v_{js},v_{k1})right) end{aligned}$$
(14)
yielding the following improved algorithm:
Initialize a vector z of length J with values (1/2^K). Then, for (i=1,dots ,N):
- 1
Compute a vector of length J by computing (L_i(h(v_{j1}))) for (j=1,dots ,J). Multiply it term-wise with z to get a vector representing ({text {Pr}}(d_1,dots ,d_i,r_i=v_{j1})) for (j=1,dots ,J).
- 2
If (i<N), compute a matrix (M^*) of size (Jtimes J) representing (sum _{s=1}^{n_j}T_i(v_{js},v_{k1})) for (j,k=1,dots ,J). Then set z equal to the matrix product (zM^*), so that z now represents ({text {Pr}}(d_1,dots ,d_i,r_{i+1}=v_{j1})) for (j=1,dots ,J).
Finally, sum the elements of z to obtain the probability we wanted to compute.
To use this algorithm, one needs to find a subdivision like the one described above. See the Appendix for an algorithm that derives the optimal subdivision. We also need to compute the matrix (M^*) for each i. In the Appendix we show that
$$begin{aligned} sum _{s=1}^{n_j}T_i(v_{js},v_{k1}) = sum _{r=0}^KA_r(v_{j1},v_{k1})p_i^r(1-p_i)^{K-r} end{aligned}$$
(15)
where
$$begin{aligned} A_r(v_{j1},v_{k1}) = #left{ v_{js}: v_{js} text { and} v_{k1} text { differ at} r text { locations}right} . end{aligned}$$
(16)
In other words, the entries of the matrix is a polynomial in (p_i), with integer coefficients found by counting the differences between inheritance pattern representatives. Note that, to any pedigree there is associated a unique such matrix of polynomials. As they may play a fundamental role in pedigree computations using Markov-modelled linkage, we believe such matrices should be studied further. We look at a number of examples in Sect.3.2.
Data, and simulation and comparison procedure
The assessment of our observation model in Sect.3.1 uses the Coriell sample NA12878, a genomic reference material (Coriell Institute) sequenced in Tillmar et al. [13] on a Illumina MiSeq instrument. Results for samples with varying amounts of DNA and varying allelic depths are shown in Table1.
The simlation uses 3929 SNPs from Tillmar et al. [13], describing a SNP panel with autosomal SNPs evenly spread across the chromosomes. Genetic positions are downloaded from Ruthers repository [22]. From Tillmar et al, we further use genotype data for the Coriell sample NA12878 to obtain coverage statistics. Allele frequencies are extracted for individuals with European ancestry (CEU) from the 1000 Genomes project [23]. We generate founder alleles through the population model in Sect.2.3 with (theta =0.01) and (gamma =0.001). We continue to drop alleles through the pedigree using the inheritance model in Sect.2.4, with crossover probabilities derived from the genetic positions alluded to above. Next, to mimic low coverage data (lcNGS) based on reduced-quality samples, we use the model in Sect.2.2 with (m=10) and (e=0.02) to generate sequence read data. The allelic depths are drawn independently for each locus using a discretized Gamma distribution, first with expectation 10 and standard deviation 2 for Figs.2 and3 and then with expectation 3 and standard deviation 1 for Figs.4 and5.
Our five example pedigrees. For each we indicate the numbering of the persons, the numbering of the parentchild relationships and which persons are tested (filled symbols)
In the simulation study in Sect.3.3 we focus on whether two persons are second cousins (see Fig.1) or unrelated. For each relationship 1000 cases are simulated and a Likelihood Ratio in favour of relatedness is computed using three different methods: Our proposed method, an amended version where linkage is ignored, and an amended version where genotypes are called. To make it optimally competitive, the calling algorithm uses the same likelihood as in our model, combining it with prior probabilities for genotypes based on allele frequencies and selecting the genotype that maximizes the resulting posterior. In other words, the called genotype is the one that maximizes the product of the population frequency of the genotype and the likelihood of the data given the genotype, where the likelihood is computed as in Sect.2.2.
For each simulated case we also estimate Jacquard coefficients using NgsRelate [18]. We use VCF-files as input, with PL-fields derived from the same data likelihoods we use in our proposed method. The Euclidean distances from the estimated point (k=(k_0,k_1,k_2)) of non-inbred coefficients to corresponding points representing the second cousin relationship or unrelatedness are computed. Comparing the difference in distances to a cutoff value yields a classification of cases into related or unrelated. Varying the cutoff value yields receiver operating characteristic (ROC) curves seen in Figs.3 and5. For comparison, the figures also show results for other methods, converted to ROC curves using the LR as cutoff.
Implementation
R-code with our algorithm is available at https://familias.name/lcNGS. To validate the correctness of our implementation, we used the software Merlin [9] which has been widely used to compute likelihoods for pedigrees and genetic data. We constructed input files for Merlin using data for a range of cases with simulated data for the SNP markers published in Tillmar et al [13]. Likelihoods were computed in Merlin and in the R script alluded to above implementing our model and subsequently compared.
Our R implementation of the pedigree preprocessing step had running times of 23s for a pedigree of second cousins on a standard 2.6 GHz laptop. A complete example computation provided at the link above, involving preprocessing and comparing 5 pedigrees, had a running time of about a minute.